Nvidia AI Releases Llama-3.1-Nemotron-51B: A New LLM that Enables Running 4x Larger Workloads on a Single GPU During Inference
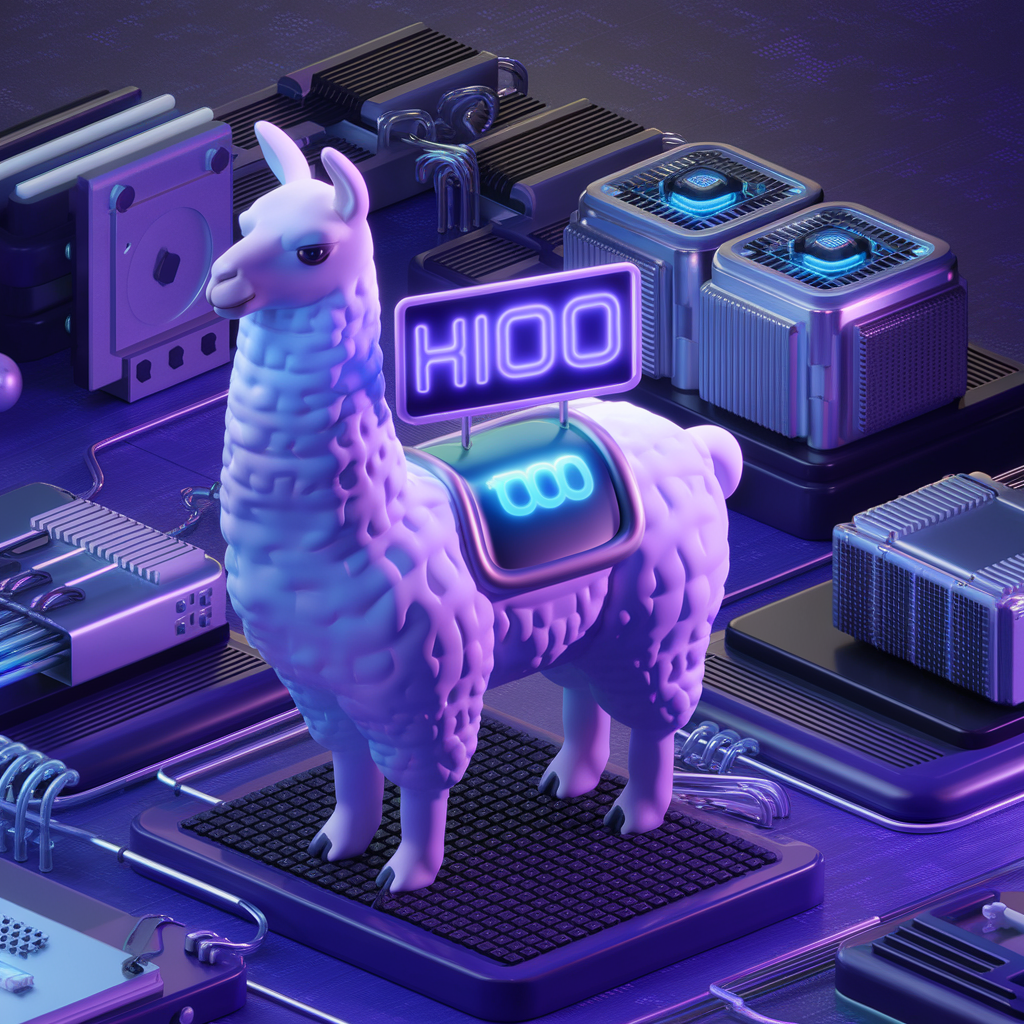
Nvidia unveiled its latest large language model (LLM) offering, the Llama-3.1-Nemotron-51B. Based on Meta’s Llama-3.1-70B, this model has been fine-tuned using advanced Neural Architecture Search (NAS) techniques, resulting in a breakthrough in both performance and efficiency. Designed to fit on a single Nvidia H100 GPU, the model significantly reduces memory consumption, computational complexity, and costs associated with running such large models. It marks an important milestone in Nvidia’s ongoing efforts to optimize large-scale AI models for real-world applications.
The Origins of Llama-3.1-Nemotron-51B
The Llama-3.1-Nemotron-51B is a derivative of Meta’s Llama-3.1-70B, released in July 2024. While Meta’s model had already set the bar high in performance, Nvidia sought to push the envelope further by focusing on efficiency. By employing NAS, Nvidia’s researchers have created a model that offers similar, if not better, performance and significantly reduces resource demands. Regarding raw computational power, the Llama-3.1-Nemotron-51B offers 2.2x faster inference than its predecessor while maintaining a comparable level of accuracy.
Breakthroughs in Efficiency and Performance
One of the key challenges in LLM development is balancing accuracy with computational efficiency. Many large-scale models deliver state-of-the-art results but at the cost of massive hardware and energy resources, which limits their applicability. Nvidia’s new model strikes a delicate balance between these two competing factors.
The Llama-3.1-Nemotron-51B achieves an impressive accuracy-efficiency tradeoff, reducing the memory bandwidth, lowering the number of floating-point operations per second (FLOPs), and decreasing the overall memory footprint without compromising the model’s ability to perform complex tasks like reasoning, summarization, and language generation. Nvidia has compressed the model to the point where it can run larger workloads on a single H100 GPU than ever before, opening up many new possibilities for developers and businesses alike.
Improved Workload Management and Cost Efficiency
A standout feature of the Llama-3.1-Nemotron-51B is its ability to manage larger workloads on a single GPU. This model allows developers to deploy high-performance LLMs in more cost-effective environments, running tasks that would have previously required multiple GPUs on just one H100 unit.
For example, the model can handle 4x larger workloads during inference than the reference Llama-3.1-70B. It also allows for faster throughput, with Nvidia reporting 1.44x better performance in key areas than other models. The efficiency of Llama-3.1-Nemotron-51B stems from an innovative approach to architecture, which focuses on reducing redundancy in computational processes while still preserving the model’s ability to execute complex linguistic tasks with high accuracy.
Architecture Optimization: The Key to Success
The Llama-3.1-Nemotron-51B owes much of its success to a novel approach to architecture optimization. Traditionally, LLMs are built using identical blocks, which are repeated throughout the model. While this simplifies the construction process, it introduces inefficiencies, particularly regarding memory and computational costs.
Nvidia addressed these issues by employing NAS techniques that optimize the model for inference. The team has used a block-distillation process, where smaller, more efficient student models are trained to mimic the functionality of the larger teacher model. By refining these student models and evaluating their performance, Nvidia has produced a version of Llama-3.1 that delivers similar levels of accuracy while drastically reducing resource requirements.
The block-distillation process allows Nvidia to explore different combinations of attention and feed-forward networks (FFNs) within the model, creating alternative configurations that prioritize either speed or accuracy, depending on the task’s specific requirements. This flexibility makes Llama-3.1-Nemotron-51B a powerful tool for various industries that need to deploy AI at scale, whether in cloud environments, data centers, or even edge computing setups.
The Puzzle Algorithm and Knowledge Distillation
The Puzzle algorithm is another critical component that sets Llama-3.1-Nemotron-51B apart from other models. This algorithm scores each potential block within the model and determines which configurations will yield the best tradeoff between speed and accuracy. By using knowledge distillation techniques, Nvidia has narrowed the accuracy gap between the reference model (Llama-3.1-70B) and the Nemotron-51B, all while significantly reducing training costs.
Through this process, Nvidia has created a model that operates on the efficient frontier of AI model development, pushing the boundaries of what can be achieved with a single GPU. By ensuring that each block within the model is as efficient as possible, Nvidia has created a model that outperforms many of its peers in accuracy and throughput.
Nvidia’s Commitment to Cost-Effective AI Solutions
Cost has always been a significant barrier to the wide adoption of large language models. While these models’ performance is undeniable, their inference costs have limited their use to only the most resource-rich organizations. Nvidia’s Llama-3.1-Nemotron-51B addresses this challenge head-on, offering a model that performs at a high level while aiming for cost efficiency.
The model’s reduced memory and computational requirements make it far more accessible to smaller organizations and developers who might not have the resources to run larger models. Nvidia has also streamlined the deployment process, packaging the model as part of its Nvidia Inference Microservice (NIM), which uses TensorRT-LLM engines for high-throughput inference. This system is designed to be easily deployable in various settings, from cloud environments to edge devices, and can scale with demand.
Future Applications and Implications
The release of Llama-3.1-Nemotron-51B has far-reaching implications for the future of generative AI and LLMs. By making high-performance models more accessible and cost-effective, Nvidia has opened the door for a broader range of industries to take advantage of these technologies. The reduced cost of inference also means that LLMs can now be deployed in areas previously too expensive to justify, such as real-time applications, customer service chatbots, and more.
The flexibility of the NAS approach used in the model’s development means that Nvidia can continue to refine and optimize the architecture for different hardware setups and use cases. Whether a developer needs a model optimized for speed or accuracy, Nvidia’s Llama-3.1-Nemotron-51B provides a foundation that can be adapted to meet various requirements.
Conclusion
Nvidia’s Llama-3.1-Nemotron-51B is a game-changing release in the world of AI. By focusing on performance and efficiency, Nvidia has created a model that not only rivals the best in the industry but also sets a new standard for cost-effectiveness and accessibility. Using NAS and block-distillation techniques has allowed Nvidia to break through the traditional limitations of LLMs, making it possible to deploy these models on a single GPU while maintaining high accuracy. As generative AI continues to evolve, models like Llama-3.1-Nemotron-51B will play a crucial role in shaping the industry’s future, enabling more organizations to leverage the power of AI in their everyday operations. Whether for large-scale data processing, real-time language generation, or advanced reasoning tasks, Nvidia’s latest offering promises to be a valuable tool for developers and businesses.
Check out the Model and Blog. All credit for this research goes to the researchers of this project. Also, don’t forget to follow us on Twitter and join our Telegram Channel and LinkedIn Group. If you like our work, you will love our newsletter..
Don’t Forget to join our 50k+ ML SubReddit
⏩ ⏩ FREE AI WEBINAR: ‘SAM 2 for Video: How to Fine-tune On Your Data’ (Wed, Sep 25, 4:00 AM – 4:45 AM EST)
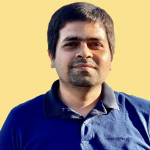
Asif Razzaq is the CEO of Marktechpost Media Inc.. As a visionary entrepreneur and engineer, Asif is committed to harnessing the potential of Artificial Intelligence for social good. His most recent endeavor is the launch of an Artificial Intelligence Media Platform, Marktechpost, which stands out for its in-depth coverage of machine learning and deep learning news that is both technically sound and easily understandable by a wide audience. The platform boasts of over 2 million monthly views, illustrating its popularity among audiences.